INTRODUCTION
IMPLAN now includes data for occupation employment, wage, and core competency (knowledge, skills, abilities, education, work experience, and on-the-job training levels for each occupation). This new data offering allows for expanding employment impact results to include occupation detail (with associated wage, education, and skill detail) in addition to Industry detail. These data are also applied to the IMPLAN study area data, providing important insights into a Region’s existing skill force, the skill requirements of various industries, and more.
This article describes the methods and sources the IMPLAN Data Team uses to estimate these occupation and core competency data.
DATA SOURCES
There are four main sources for the IMPLAN Occupation Data. The most recent data years for each are utilized.
- Bureau of Labor Statistics (BLS) Occupational Employment Survey (OES)
- BLS Employment Projections national industry by occupation matrix
- Census Bureau American Community Survey (ACS) Public Use Microdata Sample (PUMS)
- O*NET™ is a trademark of the U.S. Department of Labor, Employment and Training Administration
- www.onetonline.org
- Provides data on knowledge, skills, abilities, education, work experience, and on-the-job training by occupation.
- A new database version is released whenever updates are made.
For all sources, IMPLAN uses the most recent data release as of the time the occupation data generation process begins.
Occupation Data Year
|
OES
|
BLS
|
PUMS Data
|
O*NET
|
2018
|
2018
|
2018
|
2018
|
24.2
|
OCCUPATION EMPLOYMENT & COMPENSATION
It is important to note that IMPLAN reports occupation data only for the Wage and Salary Employment component of Total Employment, and therefore only for the Employee Compensation component of Labor Income. Note that proprietors are excluded from occupation-related IMPLAN data. This maintains consistency with Occupational Employment Statistics (OES) coverage only of “employees.”
OES data on occupation employment and wages by industry generally are available on an annual basis. IMPLAN relies most heavily on this dataset for occupation employment and compensation. The raw OES data report employment and wages by BLS SOC code, which has a hierarchy similar to North American Industrial Classification System (NAICS) codes. The SOC hierarchy occurs in 4 levels: major, minor, broad, and detail. OES data sometimes omit a level in the SOC hierarchy, e.g., reporting positive values at the detail level and its broad parent level, but nothing at the corresponding parent minor level. OES data also contain suppressions due to non-disclosure rules, with the result that a broad level code with 10 employees might report only one child detail code with 7 employees, leaving no information about the occupations of the remaining 3 employees.
When estimating occupation employment by industry, IMPLAN estimates values where that value would be non-disclosed in the OES data and ensures consistency among levels. That is, if 2 detail codes contain 5 employees each, the corresponding broad code will have 10 employees. Sometimes this requires overwriting published values that are inconsistent with other published values while ensuring that the IMPLAN data maintain as much consistency with the raw data as possible. IMPLAN’s method of disclosing missing values entails using data from aggregations at a higher NAICS or SOC level, as well as controlling lower-level values to known higher-level aggregates. Wage values are treated similarly to employment values in the disclosure process.
IMPLAN matches the disclosed and consistent occupation by industry employment data to IMPLAN Industries. In some cases, OES does not provide any coverage of an industry, such as agriculture and private households. In this case, IMPLAN supplements the OES data with data from the BLS Employment Projections, which also provide data on occupation employment by industry, but not on occupation wages by industry. Wage data from OES are substituted in this case. IMPLAN uses other BLS data to estimate military occupation employment, and deviates from the SOC system for coding military occupations. Occasionally, OES provides detail only to a NAICS level that encompasses more than one IMPLAN Industry. In such cases, IMPLAN applies the distribution of occupation employment for that NAICS code to all constituent IMPLAN Industries. Additionally, there are cases in which IMPLAN refines initial occupation estimates for an IMPLAN Industry that uses occupation data for an aggregate NAICS by accounting for a sibling industry that uses occupation data for a more detailed level of the same aggregate NAICS.
IMPLAN uses the occupation hours data from PUMS to adjust its estimates of occupation wages. The OES data on wages by occupation assume that each occupation works 2,080 hours per year (40 hours * 52 weeks), except in some cases in which it provides only an hourly wage. If IMPLAN followed that assumption, it might attribute too much compensation to occupations that tend to work fewer than 2,080 hours per year. For example, consider a hypothetical restaurant industry that has only one business. That restaurant has one waiter position, which is hired all year long but only for 20 hours per week and is paid $10 per hour. The waiter position should not be assumed to earn $10 * 2,080 = $20,800 per year; rather, the waiter would earn $10 * 2,080 * (20 / 40) = $10,400 per year. If this business also employs a restaurant manager, who works 40 hours per week and earns $20 per hour, the manager earns a total of $41,600. Properly accounting for hours worked means that the manager earns 80% of the total compensation paid by the business and in the industry, with the waiter earning the remaining 20%. Failing to account for hours worked gives the manager 67% and the waiter 33%.
CORE COMPETENCIES
KNOWLEDGE, SKILLS, ABILITIES, EDUCATION, WORK EXPERIENCE AND ON-THE-JOB TRAINING
Developed by Philip Watson, Ph.D.
Each occupation in IMPLAN is deconstructed into their constituent Knowledge, Skill, and Ability (KSA) elements as well as education levels, work experience, and on-the-job training using Bureau of Labor Statistics (BLS) data and O*NET™ data. The KSAs along with education, experience, and training are referred to as the “core competencies.”
O*NET™ data report 33 unique knowledge elements, 35 unique skill elements, 52 unique ability elements, 12 unique educational attainment elements, 11 unique work experience levels, and 9 unique on-the-job training levels. Descriptions of these core competency elements can be found in the Core Competencies spreadsheet.
In order to generate workforce reports, IMPLAN occupation data must be bridged to the core competencies. However, neither O*NET™ databases nor the BLS provides a bridge between occupations and core competencies; rather, they report values on two scales for each occupation and KSA combination. The scales that are reported are a 0-5 measure of “importance” and a 0-7 measure of “level.” Combining these two scales into a bridge of occupation employment to measures of KSA endowments requires some assumptions and empirical calculations. The assumptions used, while not intended to be regarded as the only set of assumptions that could be used to generate the bridges, were empirically tested to ensure that the resulting bridges accurately predicted occupation wages and presented a reasonable measure of the KSAs associated with each occupation.
ASSUMPTIONS
The first assumption used in generating the bridges is that the two KSA scales would be combined using a multiplicative interaction between the scales. The use of a multiplicative relationship rather than an additive interaction deemphasizes very small values for either the level or the importance and creates a higher weight for KSA elements that have higher values for both the level and the importance. Alternative interactions were also explored, including additive, arithmetic means, and geometric means, but the multiplicative interaction was found to be the best across multiple empirical tests.
The second assumption is that core competency endowments are associated with the occupations to which people are employed (or potentially unemployed) in a region rather than directly to the people themselves. Therefore when a person moves from one job to another within a region, the core competencies in the region change even though the same people reside and work there as before. More technically, the core competencies generated here are a measure of the expected human capital endowments in the region given the current occupation mix of employment in the region. This assumption relates directly to the third assumption below.
The third assumption in generating the bridge is that every occupation uses the same absolute amount of KSAs and, therefore, the differences between the KSAs associated with different occupations are in their distribution, not their level. This assumption is useful due to the incompatible units across KSA elements. The result of this assumption is that every occupation can be thought of as using 100 units of knowledge elements, 100 units of skill elements, and 100 units of ability elements in varying distributions. When a worker moves from one job to another, the worker’s mix of KSAs change, but the overall amount of total KSAs which the worker possess does not change. Likewise, under this assumption education and training do not necessarily increase the absolute level of KSAs in a region; rather, they simply enable people to move from one occupation to a different occupation with a different (and presumably more valuable) set of associated KSAs.
The chart below presents a numerical example of calculating units of knowledge elements from the O*NET data. The example is simplified, as O*NET includes many more knowledge elements for the economist occupation. According to this example, if an economy contains only one job, and that one job is held by an economist, the economy would have 100 units of knowledge, allocated among the three types listed below. The calculation is the same for other occupations and for other KSA types.
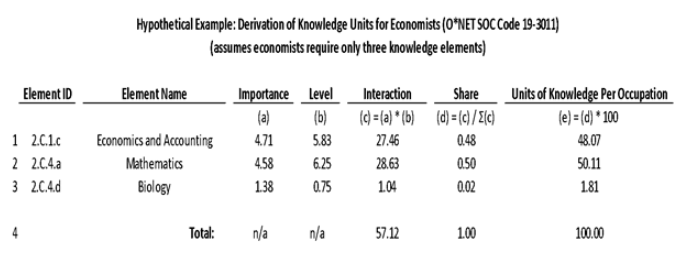
The other core competencies outside of the KSAs were more straightforward in their bridging to occupation data. Education, work experience, and on-the-job-training data from the O*NET™ database are reported as proportions of persons employed in a given occupation that each have a given level of education or training, respectively. O*NET™ data report 12 levels of educational attainment, 11 levels of work experience, and 9 on-the-job-training categories. Since they are already reported as proportions, the bridge to IMPLAN occupation data is direct and does not require any additional assumptions. The education, work experience, and training categories can also be found in the Core Competencies spreadsheet. However, similar to the KSAs, the education and training elements are occupation-based and not individual-based; therefore, they are not to be thought of as the direct endowments of the individuals in a region, but rather as the expected endowments relative to the occupation employment in the region.
While O*NET data covers the majority of OES occupations, there are occupations in the O*NET database that lack data. For example, the “all other” occupations (SOCs ending in 9: xx-xxx9) often lack O*NET data. In such cases, core competency data for the occupation is estimated using the core competency estimates of sibling level occupations weighted by each competency’s survey response size as provided by O*NET. This approach assumes that the “all other” occupation reflects the competency emphasis of the sibling occupations. Additionally, military occupations were estimated using similar means in order to bridge O*NET data to IMPLAN’s custom military occupation codes.
Given the data and assumptions described above, IMPLAN can estimate endowments of the respective core competencies which will sum to 100 times the same total as the occupation employment total in the region. For example, if there are 1,000 occupation jobs in a given region, then there will be 1,000*100 occupation equivalents of knowledge, 1,000*100 occupation equivalents of skills, and 1,000*100 occupation equivalents of abilities. These occupation equivalents will vary widely by region based on the industrial and occupation employment mix in the respective region.
DOWNLOADS
Core Competencies
Occupation SOC Codes
RELATED ARTICLES
Occupation Data Details
Using Occupation Data in IMPLAN
Occupation Data Use Cases
Occupation Data – Behind the i